In December 2020, the team at IBM published a blog post and technical paper in which they benchmarked the performance of a new-and-improved Watson Assistant against other conversational AI platforms from Google, Microsoft and Rasa.
Naturally, we were very curious.
As it happens, some of the training datasets used in the benchmark test were the same as those used in earlier research by one of PolyAI’s senior researchers, Iñigo Casanueva (You can also find a summary here). As a result, we took the liberty of adding PolyAI’s proprietary ConveRT model to the performance benchmarks for Watson Assistant, RASA and other popular language understanding models from Google, Facebook and Hugging Face.
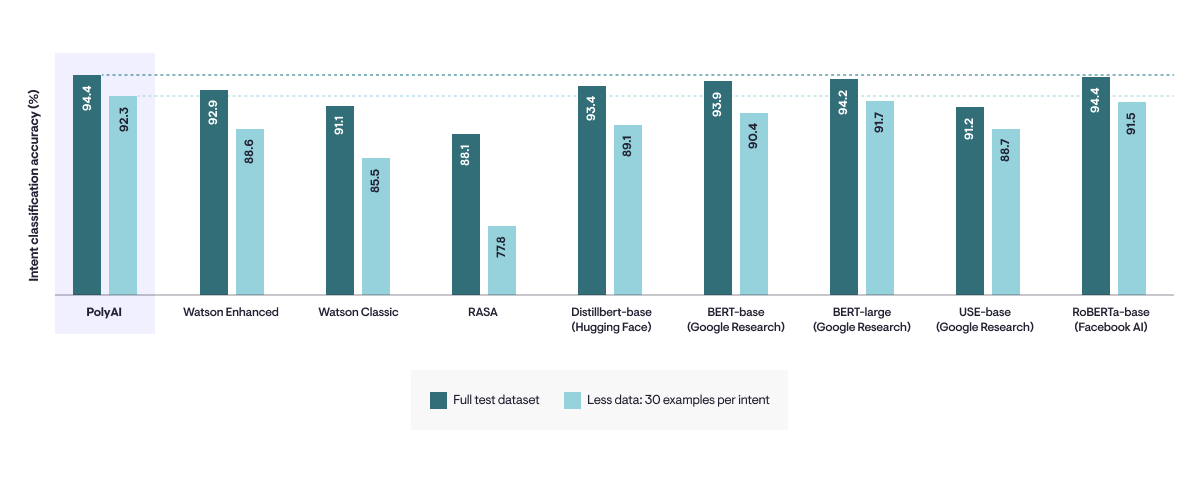
Spoiler Alert: PolyAI Remains Best-In-Class
When full datasets are used for training, PolyAI achieved an average accuracy of 94.4% – the highest level of accuracy across all vendors and a 1.5% improvement over IBM Watson Enhanced.
The full datasets include hundreds of unique examples per intent, which can be hard to collect in the real world. Thinking of, and disambiguating, hundreds or thousands of unique training examples is one of the reasons for cost blowouts and project delays when enterprises attempt to build their own voice assistants.
That’s why it’s important to also benchmark performance with less data. When training is restricted to 30 examples per intent, PolyAI achieves an average accuracy of 92.3%, the best in class. In situations with less data, our lead over IBM Watson Enhanced extends to 3.7%.
While we further evaluated our performance using only 10 examples per intent, there was no directly comparable data point in the IBM research. Needless to say, we’re fairly confident that PolyAI would remain best-in-class.
These results reinforce the findings of an independent evaluation by Salesforce Research (see here) that showed PolyAI’s state-of-the-art approach to conversational understanding outperforms the rest of the market while using only a fraction of the computation resources.
Higher Accuracy with Less Data and Less Training Time = More Agility for Enterprises
Accuracy is understanding. A higher level of accuracy means fewer frustrating interjections from a voice assistant asking customers to repeat themselves. This increases the likelihood that a customer will choose to engage with a voice assistant to solve their problems rather than wait on hold for a human agent.
Accuracy with less data and less time needed in training also has benefits for real-world applications. Customer service processes often change. The language that customers use when seeking support often reflects the latest communications they see or hear – whether via email, letters, websites or a mobile notification.
The ability to achieve accuracy with less data and shorter training times helps enterprises adapt their voice assistants, in real-time, to maintain a consistent customer experience across multiple channels. This agility ensures that business outcomes in customer satisfaction and first call resolution are resilient over time.
Let’s take 2020 for example. Companies around the world had to rapidly adapt customer service processes to reflect a new vocabulary in the middle of the global pandemic. It’s not enough to simply listen for new keywords like “COVID-19” and “coronavirus”. In banking, for example, voice assistants needed to draw associations between the language of financial hardship, government stimulus and COVID-19. When it mattered most, an entire class of natural language IVRs failed and call centres were overwhelmed.
There is a better way. PolyAI is part of a new generation of voice assistants that can learn new intents, conversational flows and even entire languages in days, not weeks or months. The recent convergence of dialogue science and deep learning has helped break traditional trade-offs between accuracy and speed.
Voice assistants that can understand better, with less training data, means:
- Faster deployment – developers spend less time crafting training examples before launch
- Quicker and easier updates – fewer training examples per intent makes life easier when it comes to updating conversational flows and troubleshooting overlapping intents
- Fine-tuning for the best experience – more computational resources can be devoted to creating the most human-like conversation and a unique brand voice for each enterprise
Request a demo today to learn more about how PolyAI helps enterprises create new conversational self-service experiences to reach new levels of customer satisfaction.